The rapid advancement of artificial intelligence and machine learning technology has led to exponential growth in the open-source ML ecosystem. As a result, building a flexible ML platform that supports best practices and interoperability with diverse offerings has become increasingly crucial for enterprises seeking to scale ML-driven business impact.
In 2022, Spotify’s machine learning platform—in active use since 2018—adopted the name Hendrix as part of our multi-year journey to develop best-in-class infrastructure for powering our ML research, products, and experiments through the complete ML journey from prototype to production.
In this talk, Mike Seid and Divita Vohra will discuss Spotify’s newly branded platform and share insights gained from our five-year journey building ML infrastructure to empower over 600 internal ML practitioners in driving ML innovation for audio-lovers around the world. We will demonstrate how designing for augmentability and customizability as first-class experiences, creating user experiences that facilitate force-multiplying end-users' work, and establishing stable interfaces are critical components of building a robust ML platform. The larger MLOps community can learn from our extensive journey building and maintaining infrastructure for machine learning and benefit from the same increases in productivity and innovation that Spotify has witnessed.
Main Takeaways:
- Enterprise ML infrastructure should be an integrated set of products that cover common end-to-end use cases and are extensible for less common use-cases. An ML platform should embrace the best practices of augmentable systems and allow extension by its tenants or teams handling ML-adjacent workflows.
- The design of ML infrastructure should remain flexible to adapt to changing requirements and challenges. This flexibility enables platform builders to integrate with various tools and technologies in an ever-changing environment. Prioritizing flexibility in design enables organizations to ensure their systems remain scalable, efficient, and effective in achieving their business goals.
Interview:
What's the focus of your work these days?
As the Product Area Tech Lead for the Machine Learning Platform, Mike’s day-to-day consists of defining the technical strategy and execution of delivery of the Product Area’s 50 person organization. Mike leads the engineering organization work to build the modern ML development experiences for Spotify Practitioners through a strong culture of collaboration, innovation and playfulness.
As a Senior Product Manager for Spotify’s ML workflows tooling and responsible ML efforts, Divita’s day-to-day is filled with discussions with ML practitioners, stakeholders in Trust & Safety, and strategic leaders to understand requirements and align ML infrastructure and responsible ML efforts with business goals. Conducting user and industry research to identify areas for improvement, emerging technologies, and regulatory requirements related to ML infrastructure are crucial tasks Divita engages in to ensure compliance while fostering a culture of learning and innovation in ML development across Spotify.
What's the motivation for your talk at QCon New York 2023?
In our 5 year journey building an ML platform at scale, we’ve tackled appropriately standardizing on TFX components for TensorFlow-based pipelines, Kubeflow Pipelines for ML workflow orchestration, and recently, Ray for accelerated ML research and development. Along the way, we have overcome numerous challenges such as user migrations, multi-tenancy, resource management, cluster versioning, observability, and effective cost-tracking, all while keeping our infrastructure aligned with larger business goals. We believe that our experience in tackling these common challenges in ML infrastructure will be beneficial to other builders and maintainers in the field.
How would you describe your main persona and target audience for this session?
- Builders and maintainers of ML Infrastructure (Machine Learning Engineers, Product Managers, Data Engineers)
- ML Practitioners leveraging enterprise ML infrastructure (Data Scientist, Researchers, Data Engineers, Machine Learning Engineers)
Speaker
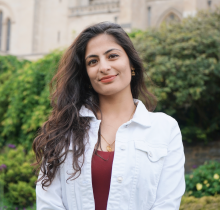
Divita Vohra
Senior Product Manager @Spotify
Divita is a Senior Product Manager for Spotify’s ML workflows and responsible ML tooling efforts. She holds a BS in computer engineering from Virginia Tech and a MS in computer science from Georgia Tech. Prior to Spotify, Divita was a PM on Capital One’s core ML platform team and served as the program lead for the Connected Circles initiative, a program designed to provide support to the women in tech community in furthering their careers in technology.
Speaker
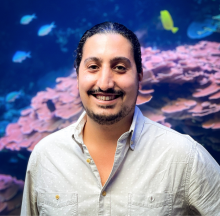
Mike Seid
Tech Lead for the ML Platform @Spotify
Mike is the Tech Lead for the Machine Learning Platform at Spotify, where he defines the technical strategy and oversees the delivery of the Hendrix built by a team of 45. His leadership is focused on driving innovation and collaboration within the engineering teams to build modern ML development experiences for over 300 ML practitioners at Spotify. By fostering a culture of playfulness and a strong sense of teamwork, Mike is driving the platform to empower practitioners to iterate on and productionize responsible ML models in an enjoyable, maintainable, and scalable way. Prior to Spotify, Mike was the Founder of Naytev(YC-S14) and an Engineering leader at Capital One, driving the delivery of a centralized feature platform to compute, serve, and register features for use in ML models.